In today’s data-driven world, businesses are constantly seeking ways to gain a competitive edge. One of the most powerful tools at their disposal is predictive analytics. By leveraging historical data and machine learning algorithms, predictive analytics can forecast future trends, identify potential risks, and uncover new opportunities. This blog explores the benefits of predictive analytics and how businesses can harness its power to drive growth and innovation.
What is Predictive Analytics?
Predictive analytics involves using statistical techniques, machine learning algorithms, and historical data to make predictions about future events. This approach goes beyond merely analyzing past performance; it enables businesses to anticipate future outcomes and make proactive decisions.
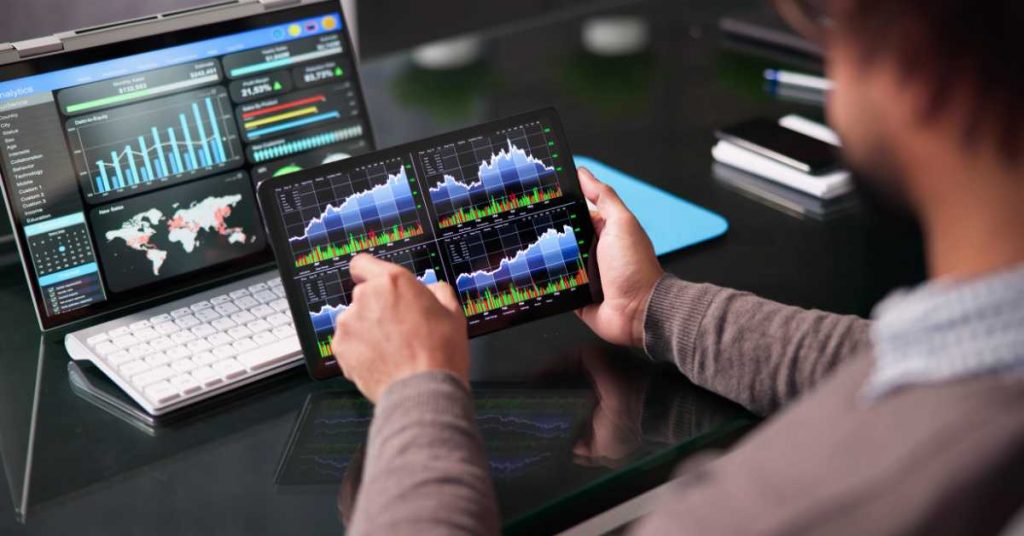
The Benefits of Predictive Analytics
- Enhanced Decision Making: Predictive analytics provides businesses with actionable insights, allowing them to make data-driven decisions. This reduces the reliance on intuition and guesswork, leading to more accurate and effective strategies.
- Improved Customer Experience: By analyzing customer behavior and preferences, businesses can personalize their offerings and interactions. Predictive models can identify the best times to engage with customers, recommend products, and even predict churn, enabling timely interventions to retain customers.
- Optimized Operations: Predictive analytics can streamline operations by forecasting demand, optimizing inventory levels, and predicting equipment failures. This leads to cost savings, improved efficiency, and reduced downtime.
- Risk Management: Businesses can use predictive models to assess and mitigate risks. For example, financial institutions can predict loan defaults, while manufacturers can anticipate supply chain disruptions. This proactive approach helps in minimizing potential losses.
- Market Insights: Predictive analytics helps businesses stay ahead of market trends by identifying emerging patterns and consumer preferences. This allows companies to innovate and adapt their products and services to meet changing demands.
How Predictive Analytics Works
Predictive analytics typically involves several key steps:
- Data Collection: Gathering historical data from various sources, including sales records, customer interactions, social media, and market reports.
- Data Cleaning and Preparation: Ensuring data quality by removing inconsistencies, filling in missing values, and transforming data into a suitable format for analysis.
- Model Building: Selecting and training machine learning algorithms on the prepared data to create predictive models. Common algorithms include regression analysis, decision trees, and neural networks.
- Model Validation: Testing the predictive models on new data to evaluate their accuracy and effectiveness.
- Deployment: Integrating the validated models into business processes to generate predictions and support decision-making.
Real-World Applications of Predictive Analytics
- Retail:
- Example: Walmart uses predictive analytics to optimize its supply chain and inventory management. By analyzing historical sales data and external factors like weather patterns, Walmart can predict demand for products and ensure shelves are stocked accordingly, reducing out-of-stock situations and excess inventory.
- Healthcare:
- Example: IBM’s Watson Health employs predictive analytics to identify patients at risk of developing chronic conditions. By analyzing patient data, including medical history and lifestyle factors, Watson Health can predict potential health issues and recommend preventive measures, improving patient outcomes and reducing healthcare costs.
- Finance:
- Example: JPMorgan Chase uses predictive analytics to detect fraudulent transactions. By analyzing transaction patterns and identifying anomalies, the bank can predict and prevent fraudulent activities, enhancing security and protecting customer assets.
- Manufacturing:
- Example: General Electric (GE) uses predictive maintenance to monitor and analyze data from its industrial equipment. By predicting when equipment is likely to fail, GE can perform maintenance proactively, reducing downtime and maintenance costs while improving operational efficiency.
- Marketing:
- Example: Amazon uses predictive analytics to personalize product recommendations. By analyzing customer purchase history and browsing behavior, Amazon’s recommendation engine predicts which products a customer is likely to buy, increasing sales and customer satisfaction.
Implementing Predictive Analytics in Your Business
To successfully implement predictive analytics, businesses should follow these steps:
- Define Objectives: Clearly identify the business goals and problems that predictive analytics will address. This could be improving customer retention, reducing operational costs, or enhancing risk management.
- Invest in Technology: Choose the right tools and technologies that align with your business needs. This includes data storage solutions, analytics software, and machine learning platforms.
- Build a Skilled Team: Assemble a team of data scientists, analysts, and IT professionals who can develop, implement, and maintain predictive models.
- Pilot Projects: Start with pilot projects to test the feasibility and impact of predictive analytics on your business. Use the insights gained to refine your approach.
- Scale Up: Once the pilot projects demonstrate success, scale up the use of predictive analytics across different areas of the business. Continuously monitor and update models to ensure their accuracy and relevance.
How GainData Enhances Predictive Analytics
GainData is a comprehensive cloud-based platform designed to help businesses overcome data-related challenges and enhance their predictive analytics capabilities. Here’s how GainData supports predictive analytics:
- Data Integration: GainData enables seamless integration of data from various sources, including customer interactions, sales records, and social media. This unified data view is crucial for building accurate predictive models.
- Data Quality: By leveraging AI, GainData ensures high data quality by cleaning and preparing data for analysis. This process removes inconsistencies and fills in missing values, ensuring that predictive models are based on reliable data.
- Synthetic Data Generation: GainData generates synthetic data to augment real data, providing sufficient data for machine learning models. This is particularly beneficial for businesses with limited historical data, ensuring accurate and scalable analytics.
- Real-Time Analytics: GainData offers real-time data analysis, allowing businesses to generate timely insights and make proactive decisions. This capability is essential for dynamic environments where quick responses are needed.
- Customizable Predictive Models: With GainData, businesses can build and customize predictive models tailored to their specific needs. The platform supports various machine learning algorithms, including regression analysis, decision trees, and neural networks.
Implementing Predictive Analytics with GainData
To successfully implement predictive analytics using GainData, businesses should follow these steps:
- Define Objectives: Clearly identify the business goals and problems that predictive analytics will address. This could be improving customer retention, reducing operational costs, or enhancing risk management.
- Integrate Data: Use GainData’s integration tools to gather data from various sources and create a unified dataset.
- Ensure Data Quality: Leverage GainData’s AI capabilities to clean and prepare the data, ensuring it is accurate and reliable.
- Build Predictive Models: Utilize GainData’s customizable predictive modeling features to develop models tailored to your business needs.
- Analyze and Act: Deploy the predictive models and use GainData’s real-time analytics to generate insights and support decision-making.
Predictive analytics is a transformative tool that can unlock significant value for businesses. By forecasting future trends and providing actionable insights, it empowers organizations to make informed decisions, optimize operations, and enhance customer experiences. As technology continues to evolve, the potential of predictive analytics will only grow, making it an indispensable asset for businesses aiming to thrive in a competitive market.
Embrace predictive analytics today and position your business for a successful and data-driven future. For more information on how to implement predictive analytics, visit GainData’s website and explore our comprehensive data solutions.